Post by naheda0011 on Nov 9, 2024 2:31:00 GMT -5
Key Takeaways from This Article
Importance of Accurate Sales Forecasting: Critical for optimizing inventory, operations, and strategic decision-making.
Data Science Methods for Sales Forecasting: Leveraging time-series forecasting, regression analysis, and machine learning for precision.
Big Data & Data Quality in Sales Forecasting: Harnessing quality data to enhance model effectiveness and demand prediction.
E-commerce Sales Forecasting Using Data Science
Introduction
Have you ever pondered the immense power of predicting whatsApp blasting your e-commerce business's sales with scientific precision? Truly effective e-commerce sales forecasting can turn the wheels of success by aligning your stock levels with future demand — an art mastered through data science.
Intrigued by the potential within your grasp? Let us guide you through a realm where numbers meet strategy, forming the bedrock of e-commerce prowess. This comprehensive guide to e-commerce sales forecasting using data science will reveal the transformative approaches that modern businesses use to drive revenue, optimize ROAS, and maximize ROI. Stay with us, and we'll unveil not just insights, but actionable strategies to propel your business into a future of data-backed abundance.
Now, what awaits you within this guide is a treasure trove of innovative perspectives and the latest trends. From the intricacies of time series analysis to the bold new horizons tapped by AI and machine learning, we're on the brink of an e-commerce revolution. The actionable intelligence you'll accumulate here could redefine your approach to market predictions and transform the way you view your data.
Prepare for a journey that promises to expand your horizons and equip you with the groundbreaking information you need to chart a course to e-commerce excellence. Let the voyage begin!
E-commerce Sales Forecasting Using Data Science
Top Statistics
Statistic Insight
Global E-commerce Sales: Forecasted to reach $6.54 trillion by 2023 (Statista). The upward trajectory shows no signs of slowing, highlighting an explosive growth in the digital market space that savvy e-commerce retailers must leverage.
COVID-19 Impact: E-commerce sales grew by 32.4% in 2020, a notable increase from 2019's growth of 14.9% (Digital Commerce 360). This spike demonstrates the agility of e-commerce in rapidly changing circumstances, proving that adaptability and data-driven foresight are essential.
AI in Sales Forecasting: AI-driven forecasts predict a potential improvement of 20-40% over traditional methods (McKinsey & Company). Businesses investing in AI are poised to gain a significant edge in prediction accuracy, leading to more precise inventory management and better customer satisfaction.
Predictive Analytics: Predictive analytics market growth projected at 22.3% CAGR from 2020 to 2027 (Grand View Research). E-commerce businesses that embrace predictive analytics can stay ahead by anticipating market trends and consumer behavior with remarkable precision.
Machine Learning in E-Commerce: The e-commerce sector's ML application is predicted to grow at a 28.2% CAGR from 2020 to 2029 (Fortune Business Insights). This statistic is a clarion call to integrate ML for enhanced customer experiences, targeted marketing campaigns, and streamlined operations.
Definition of E-commerce Sales Forecasting
E-commerce sales forecasting is the practice of predicting future sales by analyzing historical sales data and discerning patterns and trends. This predictive analysis is pivotal for making informed decisions about stocking inventory, managing supply chains, and setting marketing strategies.
Importance of Accurate Sales Forecasting in the E-commerce Industry
In the vibrant e-commerce sector, accurate sales forecasting represents a cornerstone for sustainability and growth. Precision in forecasting empowers businesses to optimize their operations, reduce holding costs, and tailor customer experiences, ultimately driving revenue and ensuring customer satisfaction.
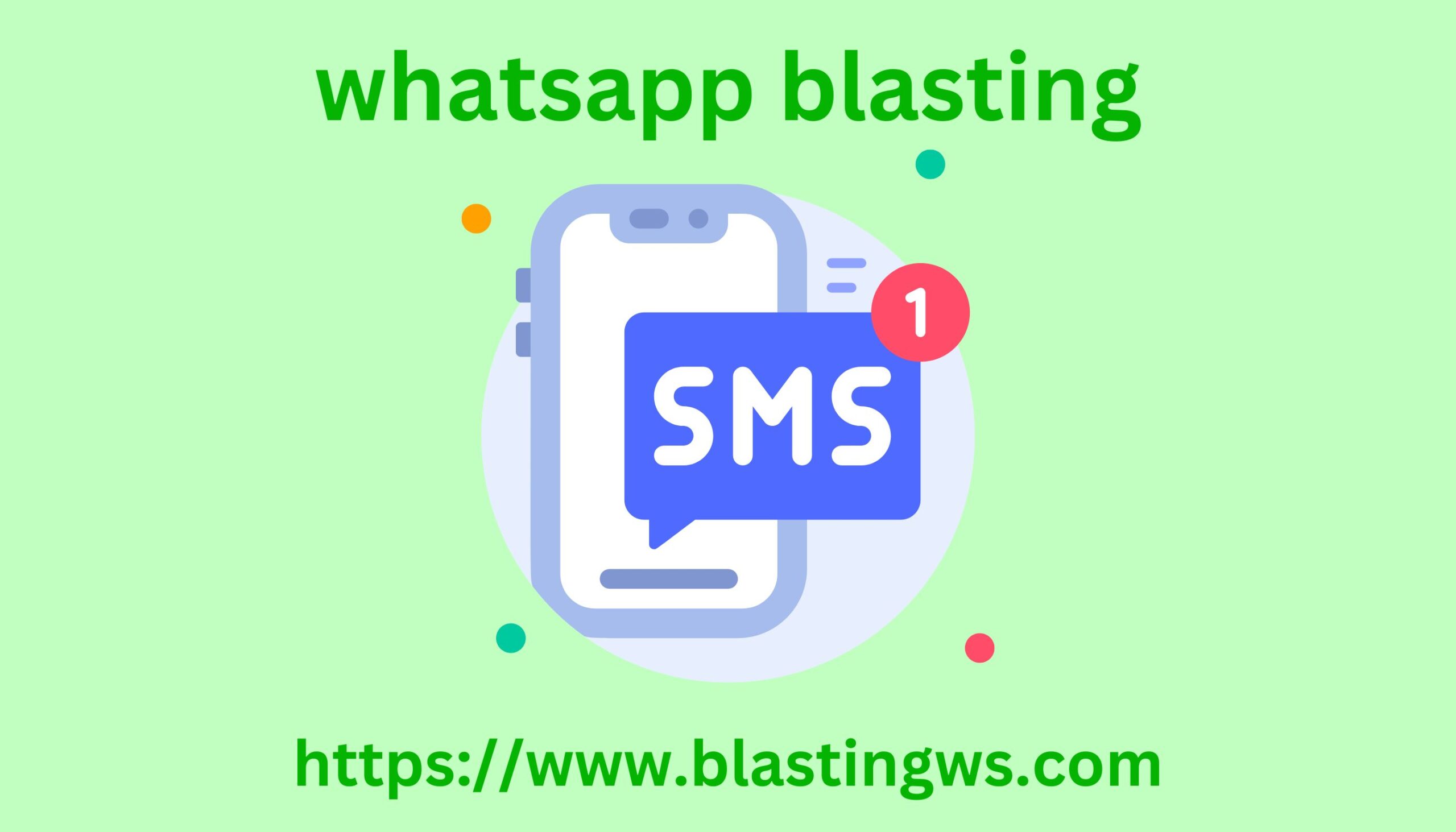
Overview of Data Science Methods for Sales Forecasting
Data science methods offer a spectrum of sophisticated statistical and machine learning tools for augmenting the quality of sales forecasts. By exploiting large datasets, these methods can uncover subtle patterns that simple projection methods may miss, leading to more precise and actionable forecasts.
Identifying Relevant Data Sources
For a robust forecast, identifying diverse and relevant data sources is crucial. Sales history, search trends, and customer behavior analytics serve as foundational elements. Moreover, external factors like market dynamics and socioeconomic indicators can provide additional perspective, creating a multi-dimensional dataset.
Data Cleaning and Transformation
Data must be cleansed and transformed to be effectively used in predictive models. This involves tackling inconsistencies, normalizing data scales, and crafting features that truly capture the dynamics of sales drivers. An impeccable dataset is synonymous with a truthful forecast.
Handling Missing Data, Outliers, and Seasonality
Mismanaged missing data or outliers can skew predictions, while unaddressed seasonality can lead to misinterpretation of trends. Effective preprocessing must address these to harness the true signal within the data, leading to a realistic forecast that accounts for cyclical sales patterns.
Time Series Analysis (ARIMA, ETS, and SARIMA)
Time series analysis, with methods such as ARIMA, ETS, and SARIMA, excels in predicting sales based on chronological data. These models are capable of capturing trends, seasonality, and even autocorrelation in sales data, providing a robust framework for making time-dependent predictions.
Importance of Accurate Sales Forecasting: Critical for optimizing inventory, operations, and strategic decision-making.
Data Science Methods for Sales Forecasting: Leveraging time-series forecasting, regression analysis, and machine learning for precision.
Big Data & Data Quality in Sales Forecasting: Harnessing quality data to enhance model effectiveness and demand prediction.
E-commerce Sales Forecasting Using Data Science
Introduction
Have you ever pondered the immense power of predicting whatsApp blasting your e-commerce business's sales with scientific precision? Truly effective e-commerce sales forecasting can turn the wheels of success by aligning your stock levels with future demand — an art mastered through data science.
Intrigued by the potential within your grasp? Let us guide you through a realm where numbers meet strategy, forming the bedrock of e-commerce prowess. This comprehensive guide to e-commerce sales forecasting using data science will reveal the transformative approaches that modern businesses use to drive revenue, optimize ROAS, and maximize ROI. Stay with us, and we'll unveil not just insights, but actionable strategies to propel your business into a future of data-backed abundance.
Now, what awaits you within this guide is a treasure trove of innovative perspectives and the latest trends. From the intricacies of time series analysis to the bold new horizons tapped by AI and machine learning, we're on the brink of an e-commerce revolution. The actionable intelligence you'll accumulate here could redefine your approach to market predictions and transform the way you view your data.
Prepare for a journey that promises to expand your horizons and equip you with the groundbreaking information you need to chart a course to e-commerce excellence. Let the voyage begin!
E-commerce Sales Forecasting Using Data Science
Top Statistics
Statistic Insight
Global E-commerce Sales: Forecasted to reach $6.54 trillion by 2023 (Statista). The upward trajectory shows no signs of slowing, highlighting an explosive growth in the digital market space that savvy e-commerce retailers must leverage.
COVID-19 Impact: E-commerce sales grew by 32.4% in 2020, a notable increase from 2019's growth of 14.9% (Digital Commerce 360). This spike demonstrates the agility of e-commerce in rapidly changing circumstances, proving that adaptability and data-driven foresight are essential.
AI in Sales Forecasting: AI-driven forecasts predict a potential improvement of 20-40% over traditional methods (McKinsey & Company). Businesses investing in AI are poised to gain a significant edge in prediction accuracy, leading to more precise inventory management and better customer satisfaction.
Predictive Analytics: Predictive analytics market growth projected at 22.3% CAGR from 2020 to 2027 (Grand View Research). E-commerce businesses that embrace predictive analytics can stay ahead by anticipating market trends and consumer behavior with remarkable precision.
Machine Learning in E-Commerce: The e-commerce sector's ML application is predicted to grow at a 28.2% CAGR from 2020 to 2029 (Fortune Business Insights). This statistic is a clarion call to integrate ML for enhanced customer experiences, targeted marketing campaigns, and streamlined operations.
Definition of E-commerce Sales Forecasting
E-commerce sales forecasting is the practice of predicting future sales by analyzing historical sales data and discerning patterns and trends. This predictive analysis is pivotal for making informed decisions about stocking inventory, managing supply chains, and setting marketing strategies.
Importance of Accurate Sales Forecasting in the E-commerce Industry
In the vibrant e-commerce sector, accurate sales forecasting represents a cornerstone for sustainability and growth. Precision in forecasting empowers businesses to optimize their operations, reduce holding costs, and tailor customer experiences, ultimately driving revenue and ensuring customer satisfaction.
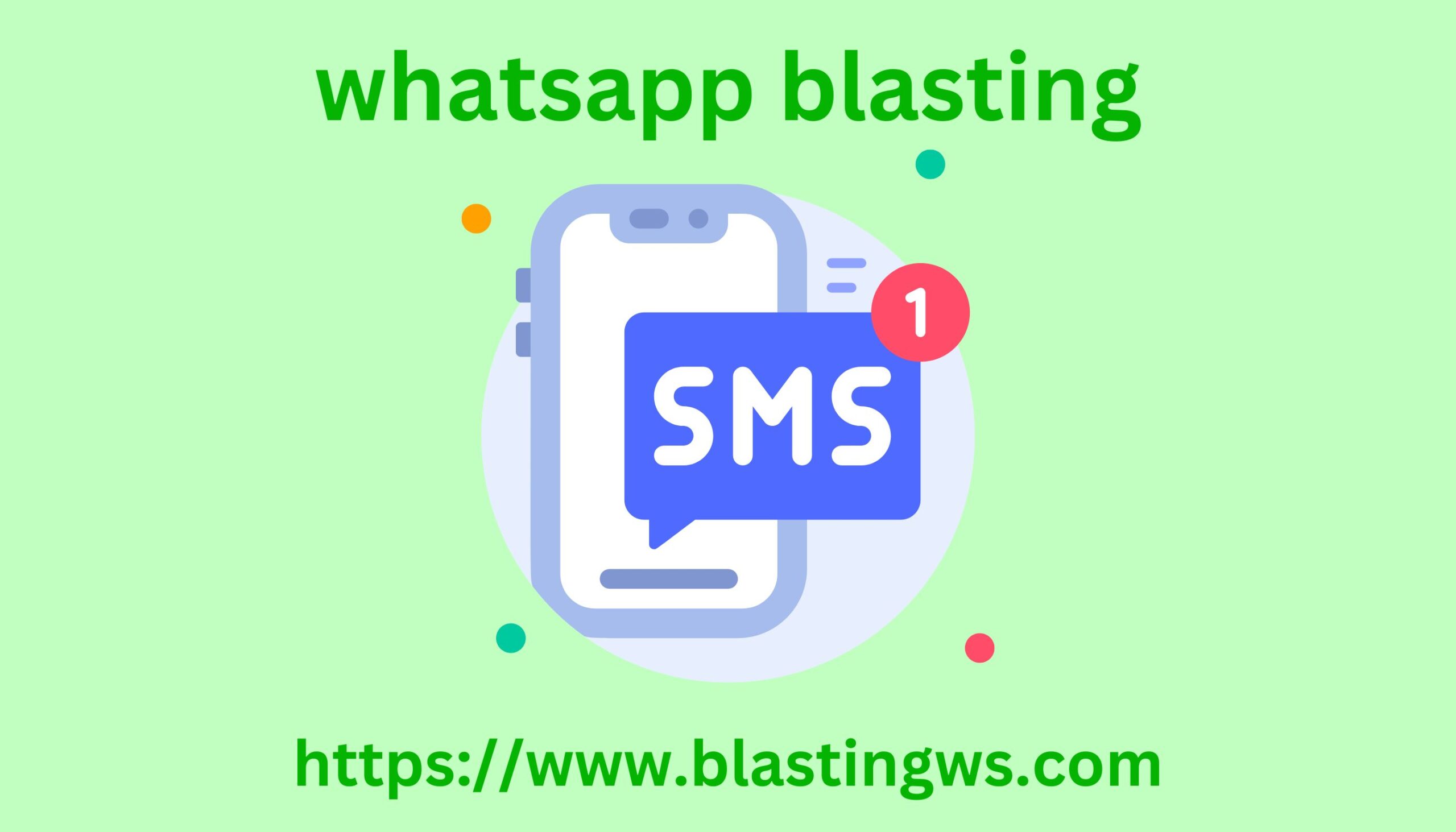
Overview of Data Science Methods for Sales Forecasting
Data science methods offer a spectrum of sophisticated statistical and machine learning tools for augmenting the quality of sales forecasts. By exploiting large datasets, these methods can uncover subtle patterns that simple projection methods may miss, leading to more precise and actionable forecasts.
Identifying Relevant Data Sources
For a robust forecast, identifying diverse and relevant data sources is crucial. Sales history, search trends, and customer behavior analytics serve as foundational elements. Moreover, external factors like market dynamics and socioeconomic indicators can provide additional perspective, creating a multi-dimensional dataset.
Data Cleaning and Transformation
Data must be cleansed and transformed to be effectively used in predictive models. This involves tackling inconsistencies, normalizing data scales, and crafting features that truly capture the dynamics of sales drivers. An impeccable dataset is synonymous with a truthful forecast.
Handling Missing Data, Outliers, and Seasonality
Mismanaged missing data or outliers can skew predictions, while unaddressed seasonality can lead to misinterpretation of trends. Effective preprocessing must address these to harness the true signal within the data, leading to a realistic forecast that accounts for cyclical sales patterns.
Time Series Analysis (ARIMA, ETS, and SARIMA)
Time series analysis, with methods such as ARIMA, ETS, and SARIMA, excels in predicting sales based on chronological data. These models are capable of capturing trends, seasonality, and even autocorrelation in sales data, providing a robust framework for making time-dependent predictions.